What’s the difference between artificial intelligence and machine learning?
What is artificial intelligence (AI)?
According to Gartner, AI applies advanced analysis and logic-based techniques, including machine learning, to interpret events, support and automate decision-making, and take action. In essence, the concept of AI centres on enabling computer systems to think and act in a more ‘human’ way, by learning from and responding to the vast amounts of information they’re able to use.
AI is already transforming our everyday lives. From the AI features on our smartphones such as built-in smart assistants, to the AI-curated content and recommendations on our social media feeds and streaming services.
What is machine learning?
As the name suggests, machine learning is based on the idea that systems can learn from data to automate and improve how things are done – by using advanced algorithms (a set of rules or instructions) to analyse data, identify patterns and make decisions and recommendations based on what they find.
Machine learning is a subset of AI , as is deep learning or neural networks, not something different. They’re all algorithms.
People used to say that information is power, but that’s no longer the case. It’s analysis of the data – the use of the data, the digging into it – that is the power.
Artificial intelligence versus machine learning
The terms artificial intelligence and machine learning are often used interchangeably, but it’s more accurate to see machine learning as a key enabler of AI. Daniel Faggella, Head of Research at AI research and advisory company Emerj, suggests it’s useful to see AI as ‘the broader goal of autonomous machine intelligence and machine learning as the specific scientific methods currently in vogue for building AI’.
What is ‘supervised’ machine learning?
Supervised machine learning algorithms are designed to learn by example. The name originates from the concept that training this type of algorithm is like having a teacher supervise the whole process.
What is ‘unsupervised’ machine learning?
Unsupervised machine learning broadens the scope of AI, enabling algorithms to analyse unlabelled datasets and identify all the patterns it can find, giving you fresh insights into the wealth of knowledge hidden in your data. Many AI experts see unsupervised machine learning as a key driver of AI. As Yinglian Xie from DataVisor suggests ‘UML eliminates the limitations of pre-existing knowledge or human bias, and will therefore enable insights never before possible.’
What is ‘reinforcement’ machine learning?
Reinforcement learning is increasingly developing AI with ‘human-like’ abilities. One of the best known examples is DeepMind’s AlphaGo, a computer program that beat the world champion at Go, a famously complex and intuitive board game, in 2017. In essence, AlphaGo learned from its mistakes over and over again until it understood how to play like a human and make the right decisions to win the game.
It’s this ability to self-learn through trial and error that puts reinforcement learning at the heart of AI technologies like robotics and automation, as well as real-world AI applications, such as precision agriculture, autonomous vehicles and the kind of ultra-personalised customer experience associated with some online retail companies, including targeted recommendations and advertising.
To enable algorithms to learn from their mistakes, reinforcement learning uses positive reinforcement, by positively evaluating decisions that take the algorithm closer to its goal.
Leading data science and AI organisations like The Alan Turing Institute, see reinforcement learning as central to adaptive, real-time machine learning, where the AI application needs to interact with the vast amount of data it gathers from its environment continuously, in order to learn and carry out its function.
Superintelligence
As the ability of AI develops, so too do its potential transformative benefits and risks. The Centre for the Study of Existential Risk (CSER) at the University of Cambridge, involving technologists, academics and policymakers, sees AI as opening the door to new scientific discoveries, cheaper and better goods and services, and medical advances. But they are also exploring the possible risks.
In the near-term, concerns around AI often focus on privacy, bias, inequality, safety and security, but CSER is also focusing on the idea of superintelligence. At the moment, AI is designed for specific ‘narrow’ applications. For example, AlphaGo was designed to master the art of Go. But as AI learns and adapts more generally, outside of specific applications, it could become superintelligent or superior to human performance in many or nearly all domains.
While CSER believes superintelligence could be used positively, they are also concerned that it could significantly magnify the kind of safety and cybersecurity issues challenging the world today
The history of machine learning
How machine learning developed – trends over time
While superintelligence still sounds to many like science fiction, the concept of machine learning has actually been around for over 60 years. The idea that computer systems could learn for themselves is credited to Arthur Samuel who first used the term machine learning when he developed the Samuel Checkers-playing Program at IBM, one of the world’s first successful self-learning progams.
Big Data and the Internet of Things
Successive innovations, from the arrival of the Internet, to the rise of Big Data, the Internet of Things (IoT) and the Industrial Internet of Things (IIoT), has enabled machine learning to develop exponentially – giving computer systems an ever greater depth and breadth of data to learn from.
Deep neural networks and deep learning
Artificial neural networks, inspired by biological neural networks in animal brains, enable computer systems to learn from data, rather than needing to be programmed. The increasing depth of multi-layered neural networks has led to the development of deep neural networks and deep learning, enabling advances like speech and image recognition and natural language processing.
As Google Cloud explores in A History of Machine Learning, the first artificial neural network was created in 1943, when a neurophysiologist and a mathematician modelled a neural network with electrical circuits, to illustrate how human neurons might work. Computer scientists began applying the idea to their work in the 1950s, and by 1959 Stanford University had used the concept to solve a real-world problem and remove echoes over phone lines.
Where will deep learning go next?
Anima Anandkumar, Bren Professor at Caltech and Director of ML Research at Nvidia, believes the next decade will focus on advancing the wider deep learning environment. For example, by developing deep active learning and human-in-the-loop learning, where intelligent data collection is part of the machine-learning feedback loop, alongside self-supervision for semi-supervised learning. Hybrid deep learning models will continue to develop too, where deep learning is combined with other frameworks such as symbolic or causal reasoning.
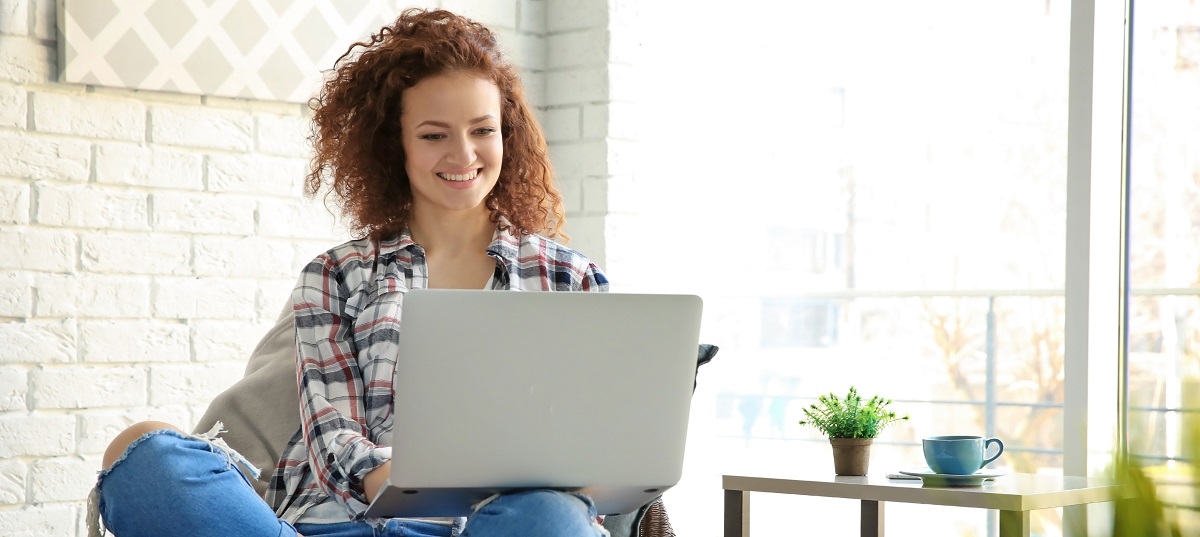
AI and machine learning – trends to watch
Mariya Yao, CTO of Metamaven and co-author of “Applied Artificial Intelligence” includes natural language processing (NLP), conversational AI such as chatbots, and computer vision among the development trends businesses of all sizes should be watching.
Natural Language Processing
NLP is the technology behind many of the online features we now take for granted including online searches and website chat boxes. A range of pre-trained language models that can be fine-tuned for specific purposes has made NLP simpler and more affordable than ever, with research now focusing on how linguistics and knowledge can be used to improve performance.
Conversational AI
Google has developed a conversational agent called Meena that can chat about anything – in contrast to traditional chatbots which are usually designed for a specific purpose. As well as making chatbot conversations sound more ‘human’ Meena represents the potential of conversational AI to help businesses gather valuable conversational data around customer preferences for example. Which in turn, will help you improve customer experience – including the customer experience delivered by chatbots.
Computer Vision
Computer vision enables AI systems to ‘see’ like humans and the technology is advancing rapidly as deep learning and artificial neural networks become more developed. In some cases, computer vision can outperform the human visual cognitive system when it comes to identifying patterns from images, by using convolutional neural networks trained to scan images pixel by pixel. As computer vision AI learns from the data it memorises, it becomes more accurate with every iteration.
We’re all familiar with computer vision AI when it comes to image captioning on social media for instance, and the technology is driving forward advances in facial recognition, biometrics, self-driving vehicles, healthcare diagnosis and fully-automated manufacturing, to name just a few of its many potential applications.
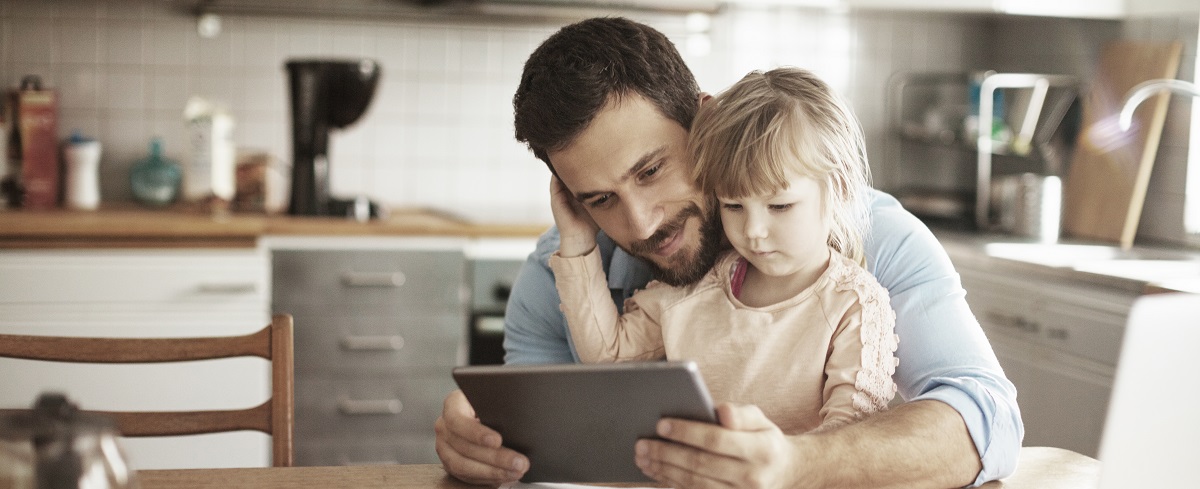
Five reasons why machine learning is important to business
In today’s society, we all leave digital footprints in multiple ways. A recent study by Aviva found that the average UK home has more than 10 internet-enabled devices, from smart TVs to remotely-operated thermostats.
These footprints offer insights into our behaviour and our likes and dislikes. This information has value to everyone, if only you can work out what it all means. And that’s where AI, machine learning and advanced analytics come in.
1.Machine learning: making the impossible, possible
Analytics has been around for decades. Many businesses are already using data and analytics to achieve their strategic goals. But when you combine analytics and machine learning you get to the complex capabilities today’s organisations need to tackle challenges and benefit from opportunities. It has the power to underpin every KPI, enrich any task, or solve any problem
2. Helping businesses to adapt and respond
Machine learning enables businesses to adapt, respond and improve their products, services and customer experiences like never before, based on a continuous flow of data revealing customer behaviour and preferences, now and in the future.
But data shouldn’t be exploited, it should be used with the customer, with their needs and wants, at the heart of it. There needs to be a clear customer centric purpose to everything
3. Improving customer experience
With machine learning and advanced analytics, businesses can make fine-tuned decisions on the spot. Businesses can respond quickly. Rather than pushing each product or service individually, it’s possible to say, ‘let’s identify the customer, understand the customer, and then make the decision.’ We’re moving from a brand or product approach to a customer centric approach. This enables businesses to have informed, relevant, personalised conversations with their customers.
For example, at Experian we’ve digitalised the whole customer journey when it comes to applying for a financial product like a credit card or mortgage. We’ve also created an engine that takes any bank statement and automatically categorises transactions, enabling organisations to accurately calculate an individual’s affordability in seconds. As well as reducing risk and opening up new opportunities, the speed of the process means customers get a fast decision.
4. Preventing fraud
Machine learning has become an invaluable tool in the fight against fraud, helping companies move from reactive to predictive by highlighting suspicious attributes or relationships that may be invisible to the naked eye but indicate a larger pattern of fraud.
The great value of machine learning is the sheer volume of data you can analyse, but selecting the right data and approach is critical. Traditionally, machine learning-based fraud detection systems have used supervised learning, which incorporates prior knowledge of fraud tactics to guide pattern identification, because it’s easy to teach the machine once there’s a clear target for it to learn.
Increasingly, many systems are also using unsupervised machine learning techniques to increase their accuracy, known as anomaly detection models. These models complement supervised learning by looking for aberrations in the patterns of a transaction flow. By combining the two together, the system can recognise previous patterns of confirmed fraud, and raise the alert if a pattern of activity changes, increasing fraud detection rates and reducing false positives.
5. Understanding customers
Machine learning will help you see things you weren’t looking for. Unlike rules based solutions like customer segmentation for example, machine learning isn’t based on assumptions, so it can help remove subjectivity and lead to more accurate, nuanced decision-making.
Making sure machine learning doesn’t reflect human biases, conscious and unconscious, is an ongoing debate and challenge for the AI community, with machine learning algorithms designed by humans and learning from human behaviour.
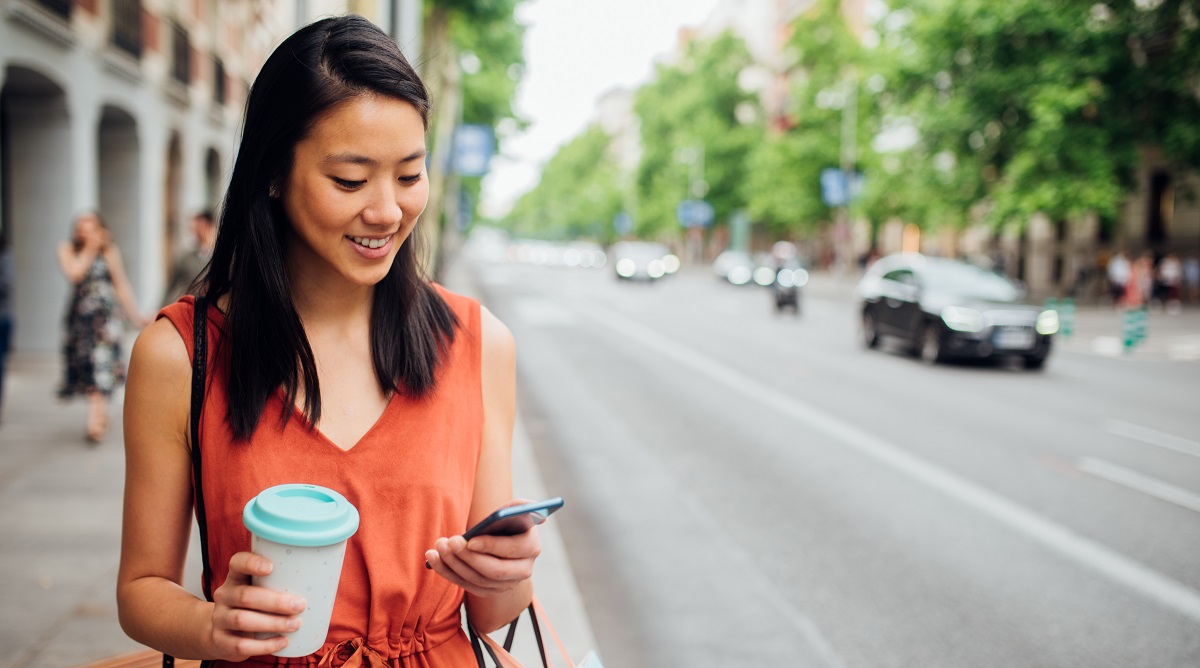
Machine learning in the financial services industry
Recent research by the Bank of England reveals how the financial services sector is increasingly using machine learning to increase efficiency, accessibility and personalisation – benefitting both organisations and customers.
More businesses are using machine learning across multiple business areas, in more in-depth ways. It’s most commonly used in anti-money laundering, fraud detection, customer services and marketing, but a growing number of companies are using it in credit risk management, trade pricing and execution, and insurance pricing and underwriting.
It’s interesting to note that the most common barriers to machine learning are organisational, from legacy IT issues to data limitations. Many firms design and develop machine learning applications in-house, but others partner with third party providers.
One potential issue flagged up by the report is that most companies apply their existing risk management framework to machine learning, but businesses are aware risk management in this area will need to evolve as machine learning becomes increasingly sophisticated.
AI, machine learning and the IoT: the challenges and opportunities for business
As the Bank of England’s report suggests, machine learning is inherently linked to data, with data limitations seen as a potential barrier to adoption. But increasingly, AI and the IoT is enabling companies to automate data gathering, as well as interpretation. The more digital devices we use more often, the more information we share with companies about our behaviours and preferences.
Good customer experience has always been key to success, but personalisation has taken this to another level. As AI advances and technologies like chatbots become able to recognise emotions and navigate complex conversations for example, it will enable organisations to gather meaningful data from every customer interaction and make sense of it in an instant.
A recent study by Futurum Research and SAS Software, suggested that the best customer experience will be delivered by a partnership of AI and humans, enabling you to optimise personalisation and improve customer loyalty and satisfaction.
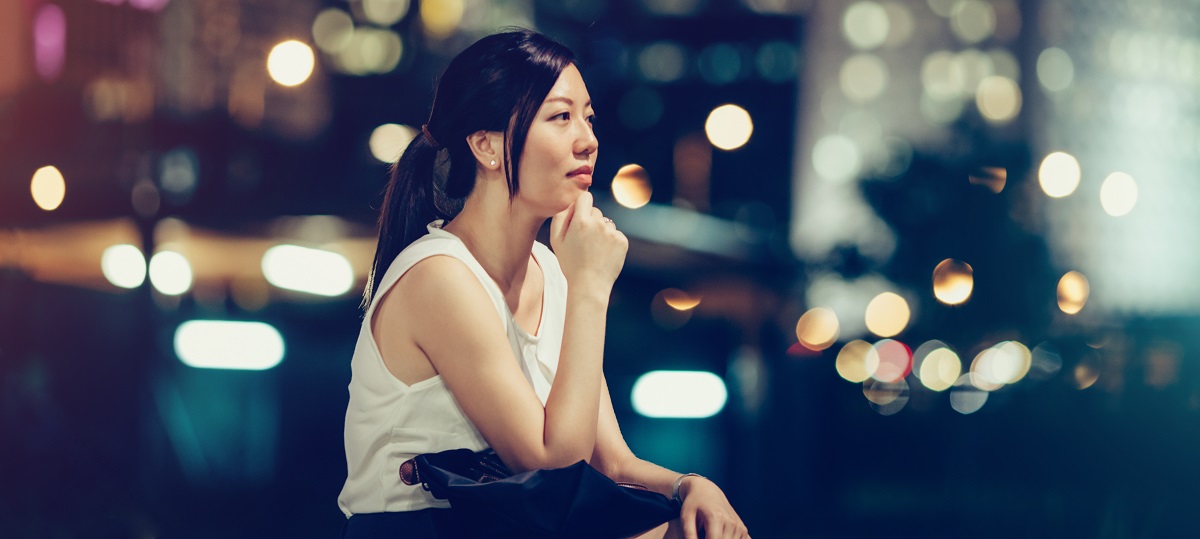
Machine learning and AI – future considerations for businesses
AI ushers in a new era of ‘intelligently automated’ data with machine learning able to iteratively improve data quality with each data update as new sources are added.
Fundamentally, every use of machine learning is reliant on data that is fit for purpose and your data strategy will need to consider the quality of your different data sources and how they work together to achieve your strategic goals.
As deep learning advances, experts predict that intelligent data collection will become part of the machine learning feedback loop, improving the way that AI models learn from the insights they generate. Similarly, hybrid deep learning combining different technologies, together with synthetic data simulations, will pave the way for machine learning in environments where data is limited.
Data privacy
As we increasingly share more data and more insights into our behaviours and preferences, it’s important for businesses to recognise the need for areas such as data privacy and consent management to keep up.
We are all, to some degree, both embracing of and sceptical about what can be done with our data. Many of us will be happy to consent to our data being used for a certain purpose, but if that purpose loses its value over time, we can quickly change our minds. How advances like the IoT and Edge AI impact risk management should be a key consideration for businesses, as the Bank of England’s report acknowledges.
The skills gap
While it’s likely that AI-driven automation could displace certain jobs, the broader AI landscape will also create new opportunities. Harnessing the right skills is one of the biggest barriers to businesses adopting AI, but that also presents a significant opportunity. The organisations who upskill their workforce around data science, machine learning and advanced analytics, alongside creative, social and emotional skills associated with human intelligence, will be the ones most able and ready to benefit from AI.
Many experts predict a rise in AI-enabled tools and bots in the workplace, focused on supporting human skills and strengthening AI-human partnerships, so it’s likely that all of us will need to learn new skills. As the physical and digital worlds become increasingly combined and smart infrastructure becomes the norm, digital transformation will also revolutionize job roles and how we work. It’s important to recognise that AI represents a paradigm shift across every aspect of our working lives, alongside the current skills gap.
Investing in AI technology
As edge computing suggests, businesses will increasingly need to replace legacy technology with new, more agile technology specifically designed to gather, store and process data, seen by many as a smart extension to cloud computing for example.
The rise of subscription-based, software-as-a-service (SaaS) products has already made automation software much more accessible for small and medium sized companies.
Next steps for businesses
To make the most of AI, machine learning and advanced analytics, you need to start from the bottom and work your way up. Our view, which is evidenced by the breadth of businesses we work with, is that working with an experienced analytics partner will help you do this. From refining how you generate, store, process and use data across your organisation, to defining your strategic goals and the insights you need to achieve them.
Ultimately, it will be your strategic goals that determine how best to invest in AI, not only in terms of technology, but how you in invest in upskilling your people and strengthening key areas such as risk management.
What are we doing at Experian?
We’ve always invested heavily in data science and advanced analytics.
In our DataLabs we have data scientists working on research and development projects globally, taking on challenges and opening up opportunities for business. Find out how our DataLabs are aiding the innovation of new products through AI and machine learning in this short video – and how we can help you manage and benefit from big data.
How do you make faster, more reliable decisions based on a depth of insight that you have never seen before? We know that the amount of resource required for a typical analytics project is vast. From the identification and access of the data sources at the start through to extracting insight and presenting it in a business-friendly format – time, money and analytical expertise are the resources you require to meet business requirements.
Experian Ascend Analytics on Demand is a powerful and secure analytics platform that gives you access to Experian’s leading consumer bureau and a range of alternative data sources, including your own, on demand. Contact us to find out how Experian Ascend Analytics on Demand can support your analytics project – from identifying and accessing the right data sources from the start, to extracting and sharing actionable insights in seconds.