In this Q&A article, we interview two of our Experian experts, Laura Hales and Junaid Zahid, to explore the challenges and opportunities of using machine learning to bring your credit scoring up to speed.
Let’s start at the beginning, what are credit scorecards?
Credit scorecards have always played a pivotal role in the success of lenders’ credit strategies. Originally, they were literal scorecards but over time the sheer volume of applications meant lenders had to move away from this approach. Today, application scorecards are statistical models developed using application data, lenders’ historical data, and third-party sources such as credit bureau data.
The most commonly used application scorecard for credit predicts the risk of a customer paying or not – helping lenders to make automated, accurate and consistent decisions on whether to approve, review or decline applicants. Beyond this, credit scoring models can help lenders predict many other metrics such as an applicant’s affordability (ability to pay) and potential future profitability. The output is usually a numeric score for each applicant, with higher scores corresponding to lower levels of estimated risk.
Why is credit scoring important, especially now?
The number one question for lenders right now is ‘how is consumer behaviour changing and what does that mean for how I make decisions?’
Credit scorecards and models give you the tools to predict how your customers will behave in the future, but the real key to answering that question is actively monitoring your models and decisions more frequently. Are your credit scorecards and models being disrupted by pandemic uncertainty or are they still working as they should be?
It’s likely you’ll need to update your credit scoring models more frequently to keep pace with the real world, by creating a set of scenarios to understand how things could play out. With models needing monitoring, recalibrating, rebuilding and deploying more frequently, lenders are also having to review their data science capabilities.
What has pandemic turbulence and uncertainty meant for credit scoring?
Traditional scorecards, which are developed using numerous variables, degrade over time. During the pandemic many of these variables have changed rapidly and drastically – including the economy, employment, income and consumer spending, savings and debt. Inevitably, credit scorecards used pre-pandemic are no longer as predictive and accurate.
As the pandemic eases, lenders will need to take the pulse of their credit scoring models more often and adapt rapidly if their model is underperforming. Lenders who paused lending due to uncertainty will need to update their credit scorecards and models now. We can help lenders gain clarity through data and analytics, and return to lending safely and confidently.
Credit scoring models are critical tools for making sound and consistent data-driven decisions. Organisations need an efficient way to monitor and optimise scoring performance and understand their performance in relation to their peers. That’s where machine learning comes in.
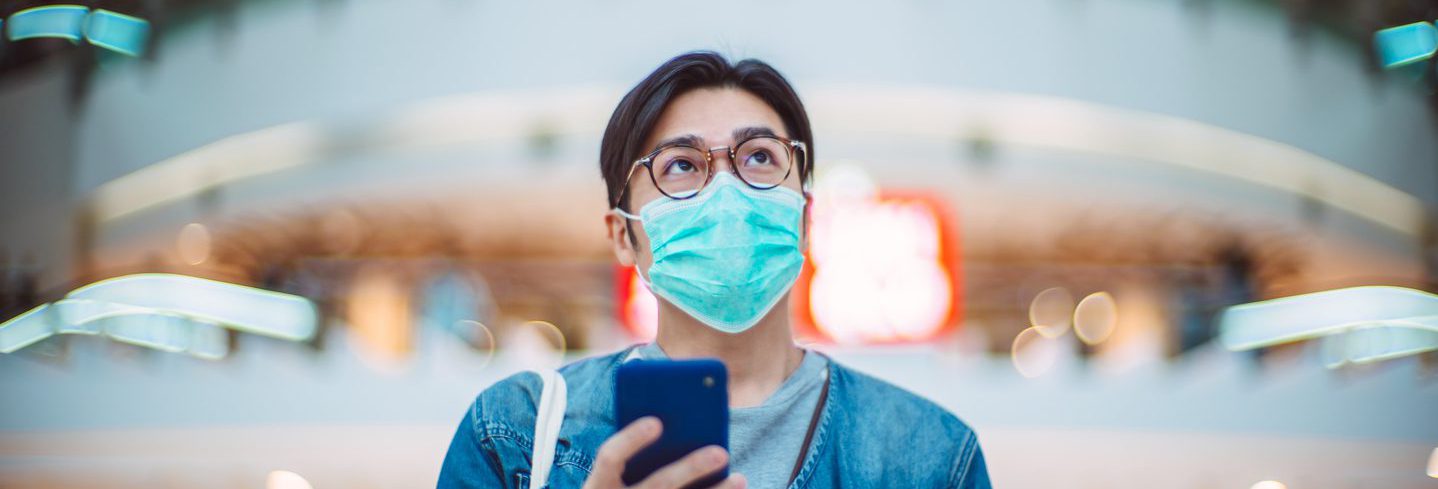
How is machine learning used in credit scoring?
Traditional credit scoring models use 10+ characteristics (such as income) to allocate points and develop a credit score for each customer. Machine learning driven credit scoring models use 200+ variables and a range of data to take a more complete view into a customer’s behaviour – so they’re much more accurate and nuanced.
Using data, analytics and technology can help you make decisions better, faster and smarter – around how you lend, what you lend, who you lend to, and how you manage your customers.
With accurate credit risk modelling needed to predict risk and value in turbulent times, we’re seeing a growing trend towards machine learning based scoring models, although machine learning is still more widely used in areas like fraud and marketing.
How will machine learning credit scoring models benefit your business?
Throughout the pandemic businesses have fallen back on data, analytics and artificial intelligence (AI) to explain what’s happening and predict what’s likely to happen next. As the UK recovers from the impact of Covid-19 and we transition to a green economy and new trading relationships post-Brexit, you will need to recalibrate or rebuild the credit scoring models you use.
Machine learning models are much faster to recalibrate or rebuild compared to traditional models, which also makes them more cost-effective. As we’ve already touched on, they’re also much more complex and advanced, and can handle a high number of variables. Enabling you to drill down into the details and interdependencies to understand your customers, predict risk and value, and spot trends and patterns.
The more accurate you can be when you lend the better. Giving the right customers the right products at the right time will drive customer acquisitions and increase your competitiveness, while lowering your risk. Using machine learning models will also help you benefit from new and alternative data sources, including open banking data (transactional level data). That kind of in-depth scoring opens up credit to consumers who may not have had access to it before, helping you to find new customers and support regulatory compliance.
How can you integrate machine learning to improve your credit decisioning process?
It’s all about finding the right approach for your business. We can work in partnership with you to take away the cost and complexity of building your own machine learning models, and the fear of taking a leap into the unknown.
Our Ascend Intelligence Services offers unique capabilities across data, analytics and AI, including advanced machine learning methods. Providing you with a fully managed analytics service designed to make the transition from traditional to machine learning models easier.
Working closely with you, our expert data scientists can rapidly build a machine learning custom credit risk model via Ascend Intelligence Services, optimise a decision strategy and deploy the model in production. Our platform has prebuilt pipelines to our vast data assets and has data wrangling capability to seamlessly combine your own and third-party data. The platform also includes our proactive model monitoring and validation service to help you monitor and validate the health of your models.
Our data scientists can help you build multiple traditional and machine learning models so you can test their performance and see the results before choosing which one to deploy – either via your own tech stack, or our Experian PowerCurve platform. Any model needs to do the same job in terms of helping you find more and better customers. It’s just that with machine learning, these models are much more accurate and faster to build and recalibrate.
We believe that machine learning and artificial intelligence tools will become much more accessible over the next few years. Training machine learning and artificial intelligence agents to accomplish specific tasks and goals, could become as commonplace as using office tools like Microsoft PowerPoint or Excel.
Improve the speed, accuracy and efficiency of scorecard monitoring and development with Experian Ascend Intelligence Services
Contact usWhat issues and trends should be on lenders’ radar now?
One of the biggest stories of the pandemic is how it’s accelerated digitalisation. Alongside businesses, consumers have become more digitally savvy and expect a quick turnaround and seamless digital journeys – online and on mobile. Our recent Global Insights Report found that 1 in 3 customers are only willing to wait for 30 seconds or less before abandoning an online transaction. In contrast, only 24% of businesses we spoke to are deliberately making changes to their digital customer journey in response. Lenders need to keep closing the gap between their digital intentions and what they actually deliver to customers.
New products and services will also put pressure on lenders to stay competitive, especially the rise of the buy now pay later sector.
What about explainability and trust?
Explainability is becoming a key focus for regulators. The EU and US is looking at specific regulation and it’s likely that the UK will follow suit. The EU’s proposed legislation covers many different aspects of artificial intelligence and specifically the use of statistical and machine learning approaches – used by lenders for credit scoring and digital marketing for example.
As the proliferation of data continues and more industries use advanced techniques to develop more predictive models, there is a growing need to explain how these new models work and verify they’re performing fairly. The lack of explanations can be a major factor that hampers the uptake of advanced machine learning models. Explainability is becoming a top priority for financial institutions making high impact decisions in regulated markets.
Ascend Intelligence Services embeds Experian’s 20 years of analytics, enabling you to build regulatory compliant, explainable models. Through our technology, we have developed a solution that aims to solve the ultimate problem of determining and fixing algorithmic and decision bias. It applies to any stage of modelling, allowing you to train, evaluate, and improve your models.
How can you assess credit scoring performance and continue to optimise?
We can help you monitor, optimise and rapidly deploy credit scoring models – saving you time and enabling you to adapt to changing market conditions more quickly. We do this by combining credit data with sophisticated machine learning models in a cloud-based platform. Model building with Ascend Intelligence Services includes an assessment of how your model will perform, and you can also monitor performance, to flag up when your model needs recalibrating or rebuilding.
We’ve been developing scorecards and models for over 20 years. With us, you’ll get access to the widest range of UK credit data, scorecard and modelling specialists, and a renowned economics team who can help you understand potential market changes and the impact on your business, portfolio or product.
We can help with customer management modelling, including strategies for managing credit limits and early warning signs to prevent customers falling into collections.
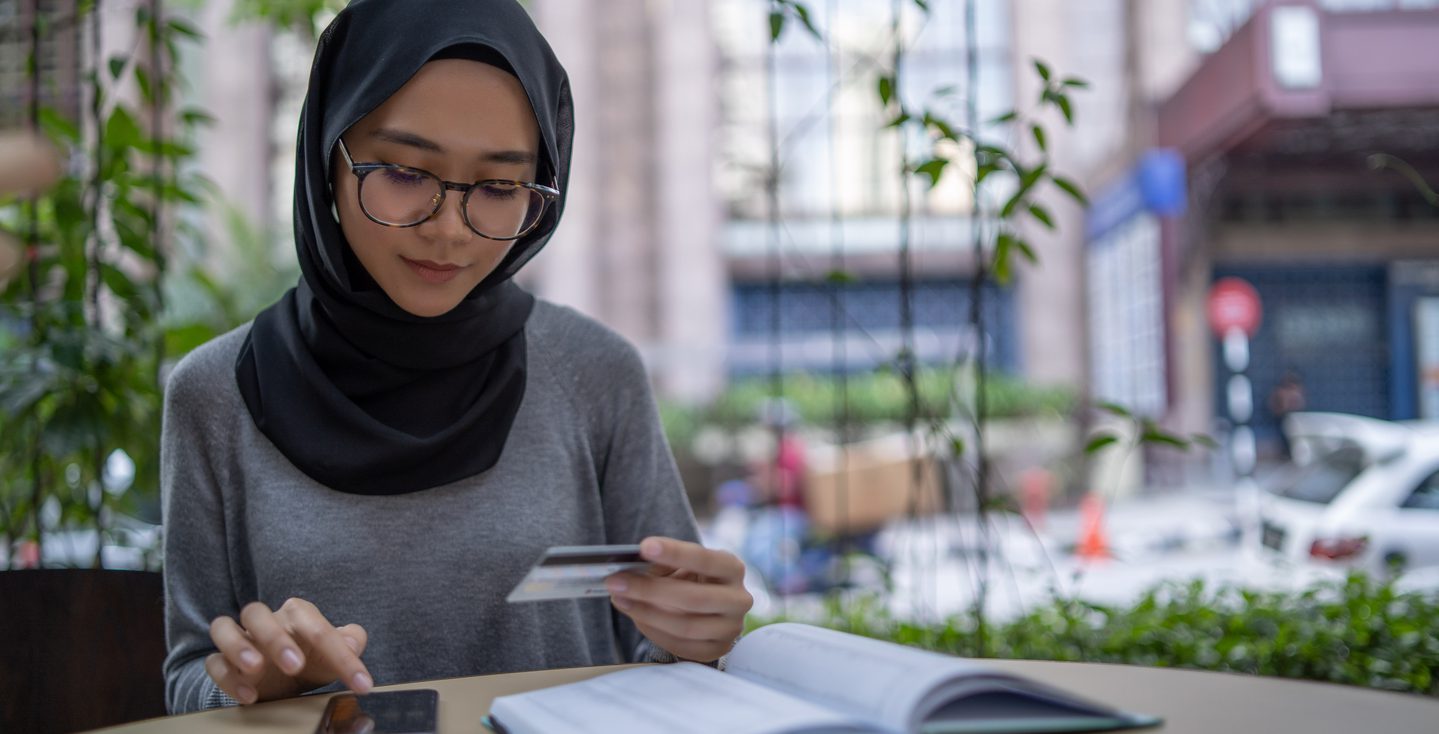
If you could offer four top tips to lenders, what would they be?
- Monitor the health of your credit scoring models more frequently
Many of the predictive models that lenders rely on aren’t stable enough to handle real-world disruptions, or recalibrated frequently enough to accurately assess risk in rapidly changing times. Monitoring models on a quarterly basis is no longer enough.
Increasing monitoring frequency and identifying when your model needs updating sooner can have a significant financial impact. Depending on the size of your business and the specific use case, lenders could save millions of pounds in lost revenue or avoided credit losses. Automating the process will help you monitor more often and support often overstretched data science teams.
- Carry out ex-ante stress testing for your models
Traditional ex-post processes are effective in simulating what would have happened historically had a new model been in place. This is an extremely valuable exercise but isn’t very helpful in the current climate of unique uncertainty and rapid change. Applying ex-ante stress testing enables you to simulate and analyse a range of possible outcomes based on changing macro conditions, evolving customer behaviours, and other uncertainties like the quality of underlying data. Giving you a forward-looking view on model performance for decisions being made right now, not just a retrospective view on decisions made historically.
- Make practical, short-term adjustments
We’ve seen in previous economic downturns that models can rapidly become unfit for purpose. There’s a strong case for making adjustments that are designed to address temporary circumstances and reviewing them at an increased frequency, rather than making changes that you expect to be robust for many months.
- Set up rapid recalibration or rebuild of models
The ability to rapidly update models will be a key differentiator as businesses compete to grow their portfolios and manage losses during and in the aftermath of this pandemic. It’s important to have the set up in place to actively work on recalibrating and rebuilding your models in a test environment, evaluate their impact, and be prepared to deploy. By increasing the frequency and efficiency of model monitoring and re-calibration, you can drive business outcomes with more impact than ever before.
How would you summarise the changes we’re seeing?
The world has changed and models need to change too. Credit risk modelling has traditionally used the past to predict the future. In a world facing multiple ongoing challenges, including pandemic recovery and climate change, the past is becoming less representative and indicative of the future. Inevitably, models will degrade faster and traditional variables won’t provide enough insight and accuracy.
Machine learning provides a fast way to build accurate models with multiple variables and data sources – helping lenders acquire more and better customers and stay competitive. In the past, scorecards were rebuilt when there was a big macro-economic change, but today’s economy is much more complex and fast-moving, with a different economic picture for different customer segments.
We’ve been building credit scorecards for decades and with machine learning we can do it much faster and more accurately, to increase your performance and competitiveness. We’re data experts that know data inside out, and machine learning experts that can help you build multiple machine learning models, fast. We can do all the data wrangling as well as the data science and model building, which means you don’t need to be a machine learning expert. We provide the expertise and through working in partnership, you’ll get better results.