The current stampede to digital transformation is an important manifestation of this – as we attempt to optimise the customer experience through the digital manifestations of our business processes with a digital manifestation of the customer themselves presented in their online identity. And those who have led these initiatives appear to be gaining early-adopter advantage according to Deloitte.
But who are my ‘customers’?
The digitization of the customer experience, and its inherent implications for fraud and security breaches, is leading to organizations taking a fresh look at what they mean by ‘customer.’ At a data level. These are no longer parties that we held partial details of the situation where most B2C businesses couldn’t even tell you the names of their customers, is fading fast. In order to fuel the fire of digital transformation – and the benefits and protections it can offer us, organisations have started to look at a fundamental level at the data they hold on customers and have decided that it is not fit for purpose. Why is this?
Unfortunately, there is no one great cause, but a multitude of issues we have known about for a long time. Each on their own was never that important to fix, but add them together and digital transformation starts to look tough. There are too many partial records and an issue of data silos: multiple systems holding customer details with differing schemas. Data is constantly ageing with no sustainable method of resolution, there are far too many duplicates, and new unverified data is arriving in real-time, all the time.
There has, of course, been much investment in ‘systems of record’ such as the aforementioned CRMs, and well as Data Warehouses, Data Governance, MDM and ODS’s and CDPs where we might hold a ‘single customer view’. But confidence in the fidelity of these systems as a basis for analytics or operations will always be questioned because they do not deal holistically with answering the question “who are my customers?”. They are all great destinations for the answer to this question, but the discipline of identity resolution is growing up to provide a framework for building robust processes that actually answer the question first.
What is Identity Resolution?
Identity Resolution is a data management process that checks, validates and appends information across devices and digital footprints using a unique matching process to create a single, data-rich profile for a person or business. It resolves customer data duplications and inconsistencies using both data management techniques and trusted reference data in tandem. Identity Resolution helps businesses to prepare, cleanse, merge or migrate data; identify marketing targets; improve analytics; prevent fraud and ensure compliance with data or sector regulations.
With particular reference to fraud prevention, Identity Resolution is a foundation stone to other identity processes such as Validation, Verification, and Authentication. Read our blog ‘What is the difference between validation, verification and authentication?’ to find out more.
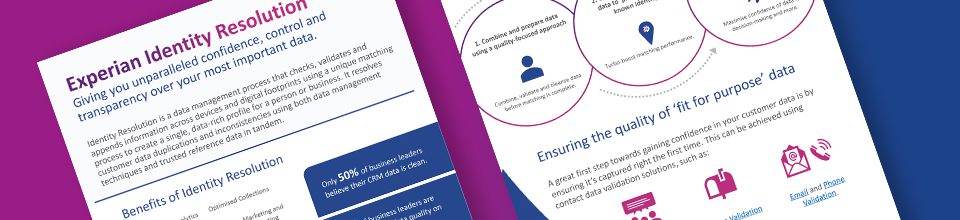
Today, businesses collect data on individuals from many different sources. This data can include:
- Web browser history
- Apps
- Customer relationship management (CRM)
- Purchase history
- Electronic transactions
- Medical history
- Previous addresses and phone numbers
- Online and offline purchases
- Social media interactions
All this data paints a picture of the personality of a person or business and can be linked to identifiers like an email address, phone number, postal address, and other contact information. This is a digital footprint of a person or business, and much like a physical footprint, all this data can be traced back to the person or business.
Early use of the term Identity Resolution concentrated almost exclusively on the process of matching and whether probabilistic or deterministic matching was superior in differencing circumstances1. Matching is, of course, important in identifying duplicates, but that is only one part of the process chain we see today.
The robust process of Identity Resolution is as follows:
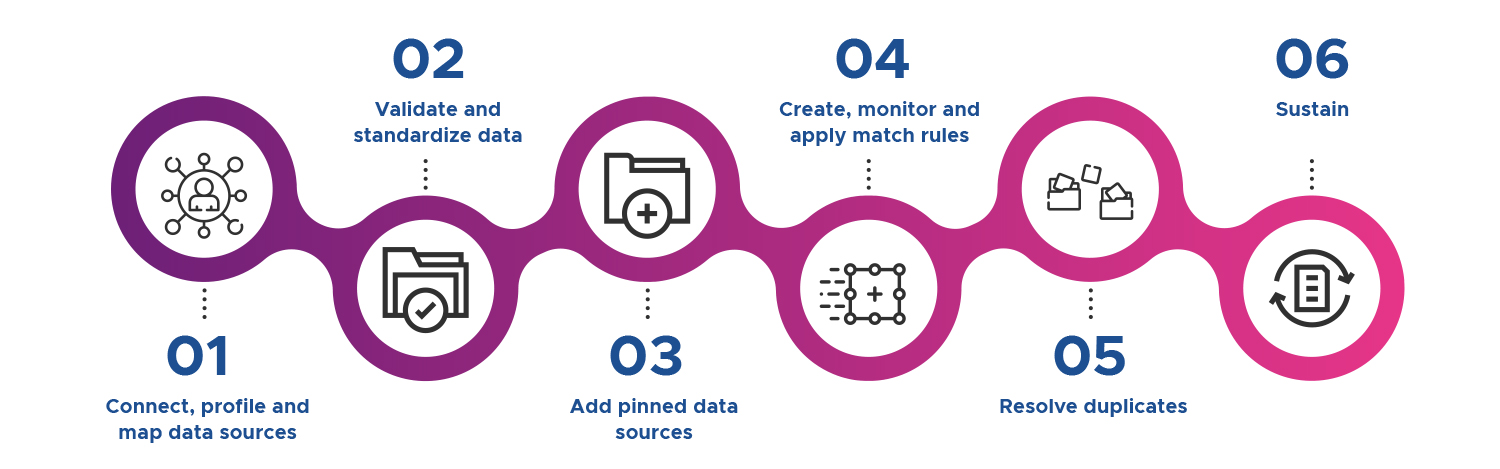
- Connect, profile and map data sources: Assimilating internal information into a common schema, with governed data rules is an important building block before we attempt to embark upon probabilistic matching. Accurately profiling what you have will reveal the potential risks further down the line. And don’t take connectivity for granted: we all love our modern data stores, but there’s still a lot of mainframe / COBOL out there.
- Validate and standardise data: Having got the data mapped, you can use simple checks to ensure that it is standardised (numbers and numbers, dares are dates and everything in in the right column and de-concatenated where needed), formatted correctly using a regular expression (regex) type masking ad then validated against external sources to ensure the accuracy and existence of things like addresses, phone number and emails.
- Add pinned data sources: We then need to think about how we can also use external sources to enrich or augment the data we have. I have used the example of pinning above, but we could also think here about adding geolocation, demographic and other data sources. Doing it here, rather than in each source system makes a lot of sense, as we will get consistent results.
- Create, monitor and apply match rules: We will discuss matching in great detail elsewhere. Just a couple of points.
- Why replace a black box matching solution from a pinning bureau with a black box solution of your own? You have no access to the bureau data records, so have to take the matches on trust. But you know your own data, so why not make the match rules transparent and easily understood by business users? This will engender trust.
- You will almost certainly need more than one set of rules. You may want identity resolution for targeting and campaign suppression lists, frequency management, insight measurement, content and offer personalisation, data sharing, and more. Allow for variation according to how much you want to trade accuracy of matching with the volume of matches you want.
- Pinned data sources (Social Security Numbers, Passport Numbers, ExPin, Company Registration Number etc.) provide useful deterministic matching, but incomplete records, ‘foreign records’, and incomplete details from source records means that they are only one input into a probabilistic matching model.
- Resolve duplicates: Duplicate resolution is not mandatory, but a golden record can have distinct advantages for analytics and mastering. But don’t throw out the other records in the cluster – they can be useful too – especially where customers continually vary how they refer to themselves – nicknames, salutations, married and non-married names are so of the complexities you need to capture when a customer turns up at your virtual door. “Do I know this person/ business?” is a question that may not be answerable just by asking them to identify themselves.
- Sustain: As mentioned, this is a process, and we need to make decisions about how often that process runs in tune with the rhythm of whatever outputs it is feeding. Overall, it is pretty safe to say that the yearly or quarterly building of customer views is long gone. Evergreening also encompasses how we handle deltas – what changed since the last time the process was run? How do we make programmatic changes within the rules we have built?
Who needs and does Identity Resolution?
The glib answer here might be that the more customers you have, the more important this becomes. But the reality is somewhat more subtle. What is truly important is the value of the use case underlying the desire to embark on Identity Resolution. At Experian, we have found that some of the pioneers in this area have come, perhaps surprisingly, from the government. As you can read at polices forces such as Cleveland and West Yorkshire the criticality is high. Beyond this, the greatest take-up has been in financial services – where the Know Your Client or Know Your Customer (KYC) legislation looms large – and then retailers, who have become so much more dependent upon their online presence. With retailers, and also gaming organizations and airlines, we have found interesting case studies around fraud, loyalty schemes and marketing.
Conclusions
Identity Resolution is the key to knowing who your customers are in a way that is fuller and more holistic than previous approaches. As your CRM slowly fills with duplicates, your customers deceive you or misinform you when they fill out your online forms, the data in your databases deteriorates in value as they move location, change name, marry and die. Further you may take on more systems and more data of the same or additional customers through M&A initiatives, or as you try to assimilate data feeds from your trading partners. As you try to do all of these things, Identity Resolution can work quietly, consistently and regularly in the background to help make sense of it all. With careful planning and an eye always fixed on the use cases to be solved, it can help deliver Clarity, Transparency and Confidence in building a single view of whomever you do business with.